Aerial Comparison
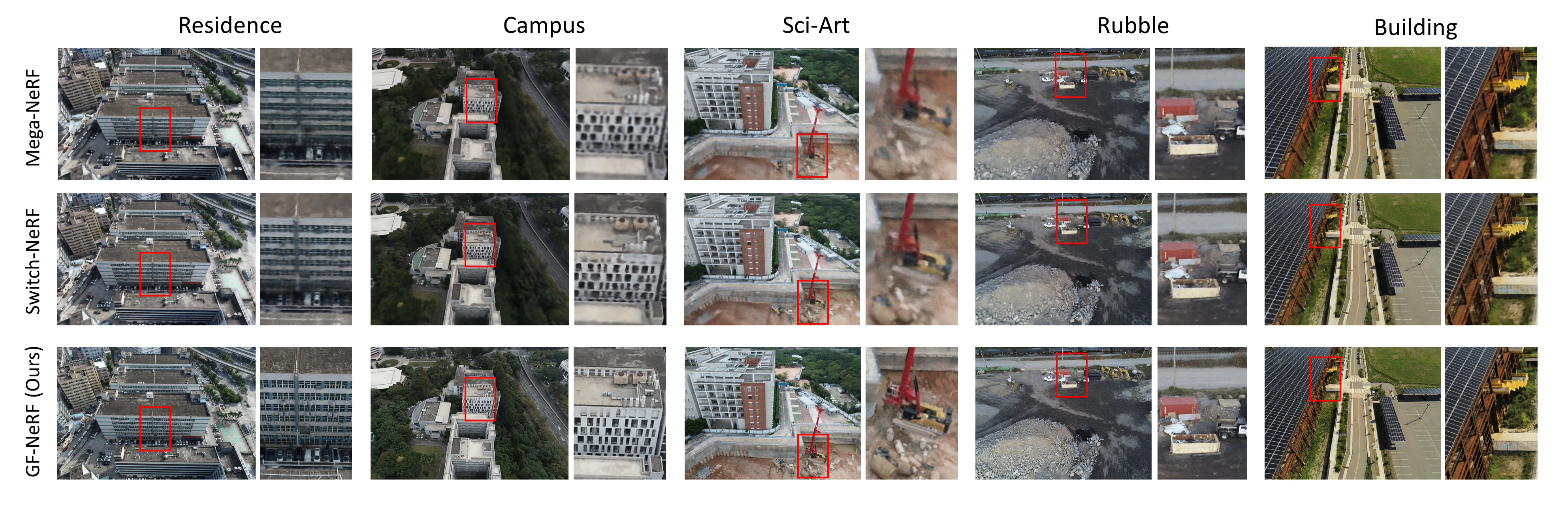
Neural radiance fields~(NeRF) have recently been applied to render large-scale scenes. However, their limited model capacity typically results in blurred rendering results. Existing large-scale NeRFs primarily address this limitation by partitioning the scene into blocks, which are subsequently handled by separate sub-NeRFs. These sub-NeRFs, trained from scratch and processed independently, lead to inconsistencies in geometry and appearance across the scene. Consequently, the rendering quality fails to exhibit significant improvement despite the expansion of model capacity. In this work, we present global-guided focal neural radiance field (GF-NeRF) that achieves high-fidelity rendering of large-scale scenes. Our proposed GF-NeRF utilizes a two-stage (Global and Focal) architecture and a global-guided training strategy. The global stage obtains a continuous representation of the entire scene while the focal stage decomposes the scene into multiple blocks and further processes them with distinct sub-encoders. Leveraging this two-stage architecture, sub-encoders only need fine-tuning based on the global encoder, thus reducing training complexity in the focal stage while maintaining scene-wide consistency. Spatial information and error information from the global stage also benefit the sub-encoders to focus on crucial areas and effectively capture more details of large-scale scenes. Notably, our approach does not rely on any prior knowledge about the target scene, attributing GF-NeRF adaptable to various large-scale scene types, including street-view and aerial-view scenes. We demonstrate that our method achieves high-fidelity, natural rendering results on various types of large-scale datasets.
@article{shao2024global,
title={Global-guided Focal Neural Radiance Field for Large-scale Scene Rendering},
author={Shao, Mingqi and Xiong, Feng and Zhang, Hang and Yang, Shuang and Xu, Mu and Bian, Wei and Wang, Xueqian},
journal={arXiv preprint arXiv:2403.12839},
year={2024}
}